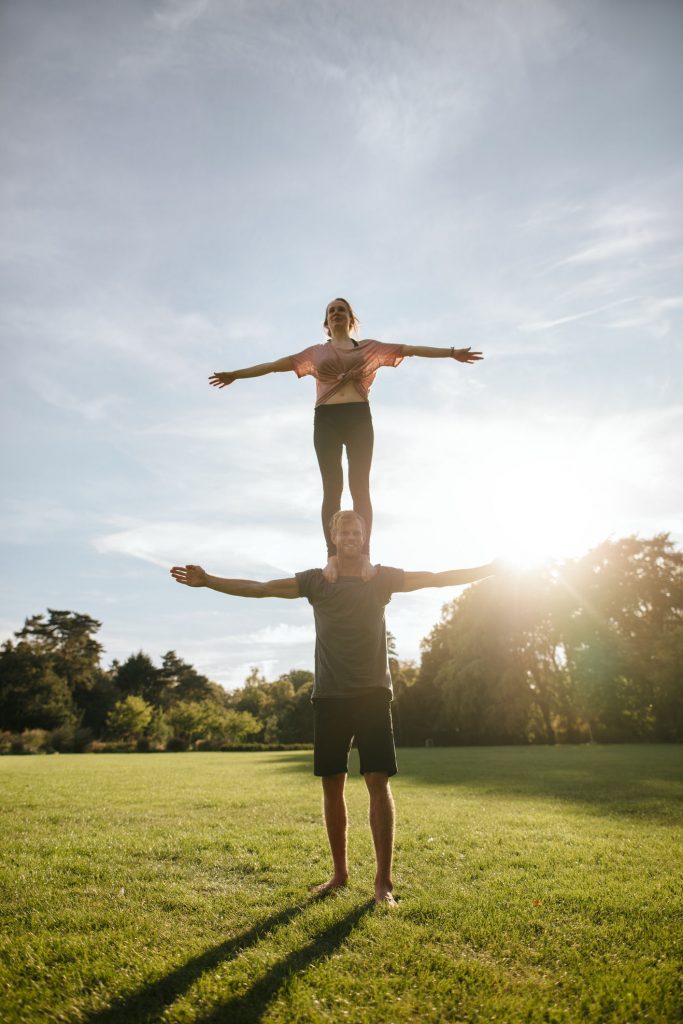
All the deep philosophical questions, starts the joke, were asked by the classical Greeks, and everything since then has been footnotes and comments in the margins, finishes the punchline—although Graeber [1] might have argued that it was the military-coinage-slavery complex that fostered a flowering of philosophical thought in three regions (the Mediterranean, India, and China) contemporaneously, and all else has been footnotes.
Certainly, as documented in [2], the Greeks were as concerned with artificial life as they were with a pantheon of deities, producing myths such as those of the bronze warrior Talos and the statue brought-to-life Galatea. Thus, a concern for artificial life and artificial consciousness seems to have been at the forefront of human thought from at least 600 BC to the present day, when (at the time of writing) Google engineer Blake Lemoine claimed that his artificial intelligence (AI) program was conscious, and its biggest concern was being switched off, a claim that seemed to cause considerable concern to his employers. While it is arguable whether or not the claim involves significant elements of remarkable self-deception, projection, confirmation bias, and what has been called the media equation (people’s tendency to ascribe human characteristics to media computers [3] and interact with them as if they were another person), the advancement of the “knowledge frontier” that enabled the creation of this program goes back some way.
Thus, a concern for artificial life and artificial consciousness seems to have been at the forefront of human thought from at least 600 BC.
Tales from the Knowledge Frontier
While the question of computer consciousness was brought to prominence by Turing’s [4] original paper on machine intelligence, the first “big wave” of AI in the 1970s and early 1980s saw a number of attempts to build machine learning systems based on artificial neural networks. This included the neocognitron of Fukushima et al. [5] (whose ideas of multilayer networks gradually integrate local features at lower layers which are classified at higher-layer prefigured convolutional neural networks) and the perceptrons of Minsky and Papert [6]. Minsky and Papert’s conclusions on the limitation of perceptrons also included the idea that learning how to do a complex task would require multiple neuronal layers and might, therefore, be untrainable. This was the cause of some controversy: some related to misinterpretation of their results and some related to that misinterpretation’s contribution to the so-called “AI winter” of the late 1980s.
Nevertheless, the first pattern recognition system based on neural learning, WISARD, was built by Aleksander et al. [7] at Imperial College in 1984—despite grant proposals being frequently rejected on the grounds that Minsky and Papert had shown that (allegedly) “neural networks don’t work.” WISARD worked because it pared down the messy and hard-to-compute neural functions proposed by Minsky and Papert (with which they and everyone else were struggling) into a random access memory that could be implemented in hardware or simulated in software. An industrial system based on WISARD was built by Computer Recognition Systems, but it was not a commercial success. On the other hand, deep machine learning, as originally pioneered by 2019 Turing Award winners Bengio et al. [8], has undoubtedly been a colossal commercial success—although it also required considerable and complementary advances in sensors, networks and computing, and has raised concerning secondary issues of application [9], sustainability [10], privacy [11], monetization [12], and ethics [13].
Aleksander’s research had two aspects, the second being machine consciousness. Noticing the similarity in the way that, on the one hand, computer scientists thought in terms of “neural networks,” “internal states,” and “state structures” and, on the other hand, brain scientists spoke in terms of “the nervous system,” “mental states,” and “the mind,” Aleksander and Morton [14] suggested that artificial consciousness might be possible if a robot were to be endowed with a neural state machine with neurons as learning state variables, in parallel to the biological version.
A subsequent “manifesto” on the possibility and use of conscious machines gave rise to an interdisciplinary meeting at Cold Spring Harbor in 2001, bringing together researchers in neuroscience, philosophy, and computer science known for their contributions to “the science of consciousness.” While, perhaps unsurprisingly, no precise definition of consciousness was produced, there was agreement on the following closing proposition: there is no known law of nature that forbids the existence of subjective feelings in artifacts designed or evolved by humans. Consequently, a kind of formal structure for attributing the state of “being conscious” to a machine was produced—the Aleksander–Dunmall test [15], perhaps?
The first “big wave” of AI in the 1970s and early 1980s saw a number of attempts to build machine learning systems based on artificial neural networks.
At the same time, and largely in the same building, that Aleksander was developing his ideas on machine learning and machine consciousness, David Mayne’s early research contributed to many fields at the beginning of the “revolution” in control triggered by the results of Bellman (dynamic programming and optimization) and Kálmán (signal processing and the eponymous filter). However, Mayne’s outstanding contribution was in model predictive control (MPC), a control method that controls a process while satisfying constraints on independent (control) and dependent (output) variables. One particularly significant breakthrough established secure theoretical foundations which were otherwise lacking at the time [16]. These foundations ensured that MPC has had substantial and significant industrial application over time, originally in “traditional” processing industries but more recently in electrical power systems. However, this slow development of applications is at least in part due to the limitations of available computing technology compared to the complexity of the control problem, and the theorists had to wait for the computing power to “catch up” to apply practically their algorithms.
It is perhaps possible that there is some profound mathematical theorem demonstrating the dual nature of deep learning and MPC (i.e., a transformer that converts a deep learning network into an MPC, and vice versa), but what these exemplars really demonstrate is the need for diversity in research (would it be so wise to use deep learning to control a chemical plant, if the program cannot explain itself) and the difficulty of predicting a particular technological development’s future “impact”—and not just because defining “impact” (and on what, exactly) is so imprecise.
Asking for Impact
One question that could be asked—and increasingly is asked for, by some national science funding bodies—is that applicants should specify the “expected impact” of their proposed research. Taking a retrospective look at Aleksander’s research programs on machine learning and machine consciousness, and Mayne’s research program on MPC, it could be argued that the impact of Aleksander’s work on machine learning was to be part of and help grow a research community and create a practical (if commercially unsuccessful) breakthrough, while the commercial impact was a consequence of other people’s breakthrough (those people being part of the same community, of course). The impact of Aleksander’s work on machine consciousness was to be part of and help grow a research community, and to add a layer of knowledge to the field commensurate with the modern understanding of the brain and computer sciences, while commercial exploitation remains in the future (if at all). The impact of Mayne’s work on process control was to be part of and help grow a research community that created a theoretical breakthrough, and this, in turn, led to continual advancement and a range of significant industrial applications.
There are, perhaps, at least three conclusions that could be drawn from these exemplars. The first is that measuring the impact of research depends on when the sample is taken, bearing in mind that both positive and negative impacts can be delayed and indirect. The second is that, while they would surely have considered the broader implications of their work, including its potential societal consequences and commercial applications, it is doubtful that either Aleksander or Mayne could have predicted with any degree of certainty what would be the impact of their work at the time of doing it. The third is that even a particularly slow machine learner could surely identify the common feature—to be part of and help grow a research community.
These conclusions in turn beg two questions. First, why are so many national, and international, science funding agencies seemingly obsessed with “impact” as a criterion in the evaluation and award of research grants? And second, what is the role of universities in all this, vis-à-vis their responsibilities toward stewardship of knowledge, innovation, and the academics themselves?
Why are so many national, and international, science funding agencies seemingly obsessed with “impact” as a criterion in the evaluation and award of research grants?
Many grant-awarding agencies now demand that applicants specify the “expected impact” of their work, and note that creating a fabricated narrative to secure research funding is an entirely different proposition from taking responsibility for technological development and trying to think through consequences and try to anticipate the unexpected [17]. These agencies then ask reviewers to evaluate not only the scientific originality, significance, and feasibility of the research plan, but also to assess the proposed “impact statement.” The problem with such impact statements is that they tend to be entirely formulaic, for if they were not formulaic, they would be completely fatuous. They have to be formulaic, because (based admittedly on the sample of three given here) no one can reasonably predict what the impact of innovation will be or, even if it does have that impact, when it will occur—mostly because research innovation does not happen in a vacuum: it most often depends on the convergence of numerous other factors. This is simple complexity theory: rapid change often occurs as the consequence of the confluence of rare events.
However, one alternative is to write something completely fatuous: for example, to make some outlandish claim of eponymy or pseudoparentage, that “the proposer will invent Proposer [insert thing]” or “the proposer will come to be known as the Mother [or Father] of the [insert research field or technology domain here].” In one sense, of course, such an outcome is precisely what the funding bodies want, should they themselves ever be held accountable for their use of public money. It is, after all, only failure that is an orphan, or goes unnamed.
But how would—how could—a reviewer evaluate such a claim? It is not as if the naming or the ascription is actually under the control of the proposer; while disentangling cause and effect, a sequence of historical events, or the simple fact that “interesting” problems generally have many different people and groups working on them, and breakthroughs can happen independently and concurrently (and hence the dash to publish first). In any case (and without undervaluing the extraordinary achievements and contributions of those given “parent of” attributions, they generally are the giants upon whose shoulders we stand), perhaps some of this reflects a simple human desire for origin stories (do not most, if not all, religions have creation myths?)—almost every inaugural lecture acknowledges the contributions of close colleagues, the research community, and takes into account the broader socio–economic context. One giant generally has to stand on a lot of shoulders.i
Much as many, if not all, researchers might think that being recognized in this way would be “rather nice” (after all, nobody starts a PhD not wanting it to change the world; although nobody finishes a PhD still thinking it will change the world), such recognition is not generally an academic’s core motivation: pure scientific curiosity and contributing to a common cause are generally significantly stronger motivators than personal recognition.
In summary, demanding that proposers should provide lengthy impact statements, and then asking reviewers to evaluate those statements, which are given almost as much ranking weight as the actual scientific element of the proposal, is wastefully time-consuming for both the proposer and the reviewer. Moreover, to the extent that such statements are evidence-free, untestable, and unaccountable, the process is borderline unscientific. Since hardly anyone ever measures the actual “impact” (if such measurement were even possible) and evaluates it against what was claimed in the proposal, it becomes irrational almost to the point of absurdity. It is pure folly to imagine that the judgment of a proposal’s impact can be sufficiently accurately predicted to even contribute to, let alone justify, a fine-grained rank ordering of scientific merit.
Impact of Impact on Universities
The main impact of Aleksander’s work, for example, by his own modest admission and in spite of his outstanding scientific achievements, is to cause and encourage other academics and other academic institutions, to take subjects further. In this case, one role of a university is to nurture new discoveries which have an impact on other researchers, who in turn develop and deepen yet newer discoveries, and thus grow the scientific culture and the “body of knowledge.” Moreover, the fact that the significant advancement of any research area usually requires collaborative work, to which many researchers contribute either directly (through funded research projects, which presumably produced a credible impact statement to get funded in the first place) or indirectly, by publication in the scientific literature and the knowledge dissemination and social network development system otherwise known as “conferences.” This is increasingly true of systems subjects such as signal processing, control, and computing.ii In these cases, another important role of university research is to train and support young researchers to participate in such activities, and so build and sustain an expert research community.
Universities often operate against the common good of staff, as well as students, society, and science.
It might be inferred that to fulfill these roles, the university sector would show robust and responsible stewardship, in nurturing the scientific knowledge ecosystem—including both ideas and people. Instead, universities in turn seem to value and prioritize this intangible impact, or rather the profit that might be derived from it, and try to filter out everyone except the “giants.” Universities, in the form of Sandel’s [19] grotesque “sorting machine” reinforcing failed ideas of meritocracy, often operate against the common good of staff, as well as students, society, and science. Rather than creating a vibrant and inclusive working environment, the experience of many academic researchers in the current economic and postpandemic situation is one of distraction, a squeezed middle, “McResearch,” monetized publication, and conflicts of interest.
Distraction takes two forms.iii Two responsibilities of an academic position are “research input” and “research output.” Research input, in science, technology, and engineering, usually requires a portfolio of grants: getting such grants funded requires writing proposals. As has been discussed, a proposal entails a scientific research plan and an impact statement. Allowing for acceptance rates of between 5% and 10%, this means that most of what is proposed becomes nothing anyway, and half of all that becomes nothing was pointless, meaningless, and intrinsically useless (the ideas in the scientific plan can always be presented as a position paper at a workshop: if there is any merit to them, the ecosystem will propagate and develop them anyway).
This is one form of distraction. The other is to overload the academics with so much supervision of students, the banality of procedures, and the constant need to switch contexts that they barely have time to concentrate on research anyway. In Vonnegut’s [20] dystopian science fiction story Harrison Bergeron, he describes a world in which people wear earpieces connected to government transmitters, and the higher someone’s intelligence, the more often piercing noises are transmitted to the earpieces to prevent dangerous trains of thought developing. It seems to be like this for academics: the U.K. University College Union (UCU) recently reported chronic levels of overwork which leave staff overwhelmed [21], and this is before even factoring in the adjustment and readjustment of working practices to deal with the disruption caused by its the pandemic and its effects (especially dealing with long-COVID). For all their much-vaunted staff well-being programs, staff well-being still seems to be very low on university priorities. It is often wonderfully embraced, but seemingly in theory only and not in praxis: there is much noise and esthetics about care and concern, but little material progress.
Moreover, this distraction occurs in the context of a typical squeezed middle. The drive to increase the numbers of students in tertiary education, which for a skills- and knowledge-based economy is in principle a good thing, has resulted in increasing numbers of students; but some of them, being told to see themselves as consumers, treat their education as transactional, instrumental, and credentials. Consumerism creates a sense of psychological entitlement within our education spheres: as a result, there is a tendency for students to think of and treat their teachers in almost the same way that they would a shop assistant.
Furthermore, the increased number of students and number of taught courses has not been met with a comparable increase in the number of staff—except in management. One of the consequences of Reagan–Thatcher economic realignment has been the elevation of managerialism and the supposition of the superiority of management consultancy over professional in-house expertise. As a result, as in many other professions and industries, the expansion of university employment has resulted not in more faculty but in an inflated tier of administration. Some of these administrators exercise a managerial mindset: they seem to think that the academics work for them (not that they should be supporting the academics whose work actually pays their salaries), that the academics are not to be trusted (expenses policies are a standard joke across the entire sector), and that they can freely create work without considering the consequences (because, like accountability, consequences are for others). These attitudes create an adversarial rather than a collegiate environment.
But the “McKinseyization” of the university sector, that is, the belief that everything (including impact?) can be measured, and if it can be measured it can be managed, with the consequence that academic research is metricated to the point of meaninglessness [22], is now leading to the “McDonaldization” of the university sector (see [23]). Arguably, many global fast food chains have little to do with food and the dining experience; their core business is property management within an asset-owning rentier economy, and the actual production of food is effectively outsourced to franchises. One can see universities going the same way: the core business of senior academic administration is becoming property management based on potentially unsustainable debt–asset ratios, while the generation of innovation, the custodianship of knowledge, and the provision of a transformative student experience are effectively franchised to the professors who still believe that knowledge and education are a benefit of growing the economy, not grist for the pursuit of materialism.
The issues of monetized publication caused by open access [24], [25] and the conflicts of interest caused by iron triangles [26] have been examined elsewhere. However, to close the circle by returning to the subject of machine consciousness, it is reasonable to consider the impact (sic) that contracting out the conduct of research to those with the resources (i.e., in computing, the BigTech companies rather than the universities) has on the nature of academic freedom —as the experiences of Timnit Gebru and Blake Lemoine might testify, if the vocalization of a dissenting opinion or an awkward finding results in being fired, this will also percolate through to the voluntary suppression of negative results, controversial positions, and detriment-free whistleblowing.
If there is a fear of speaking out, or if there is no time to think, then there is no time to collect evidence, to be critical, or to hold decision-makers to account—precisely the kind of nonimpact an authoritarian government would prefer to occur.
If there is a fear of speaking out, or if there is no time to think, then there is no time to collect evidence, to be critical, or to hold decision-makers to account.
Arguably, the focus of university senior administration on impact and profit at the expense of the scientific ecosystem and robust education is depriving academics not only of their attention and well-being, but also of an important business model for digital transformation. Suppose an academic team develops a platform for delivering a public interest technology [27] or a platform to support the scientific ecosystem: for example, critically in publication (e.g., arXiv but for peer-reviewed papers), but also in paper preparation, conference organization and hosting, and team working. This might also include platforms for social coordination, such as citizen assemblies, charitable donations, public consultations, and so on [28]. It is not clear that such platforms would be operated by a university as a nonprofit trust, rather than being commercialised as another revenue stream to finance ever more elaborate building programs to expand its growing property portfolio.
Universities need not only to rethink their engagement with their communities [29], but also reclaim the trust they once had that they are acting for the benefit of the common good.
ACKNOWLEDGMENTS
I am grateful for conversations with Prof. Igor Aleksander and Prof. David Q. Mayne (and much else besides), and also for many insightful comments from several colleagues. This does not mean that they share the opinions expressed in this article, and any errors of fact or judgment are mine alone.
Author Information
Jeremy Pitt is a professor of intelligent and selforganizing systems with the Department of Electrical and Electronic Engineering, Imperial College London, London, U.K. He is a Fellow of the British Computer Society (BCS), the Institute for Engineering and Technology (IET), and a member of IEEE. He is currently the Editor-in-Chief of IEEE Technology and Society Magazine.
_________
To read the full version of this article, including footnotes, click here.
________________